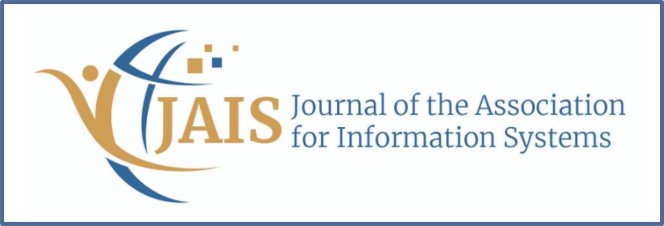
Abstract
Machine learning (ML) models often endogenously shape the data available for future updates. This is important because of their role in influencing human decisions, which then generate new data points for training. For instance, if an ML prediction results in the rejection of a loan application, the bank forgoes the opportunity to record the applicant’s actual creditworthiness, thereby impacting the availability of this data point for future model updates and potentially affecting the model’s performance. This paper delves into the relationship between the continuous updating of ML models and algorithmic discrimination in environments where predictions endogenously influence the creation of new training data. Using comprehensive simulations based on secondary empirical data, we examine the dynamic evolution of an ML model’s fairness and economic consequences in a setting that mirrors sequential interactions, such as loan approval decisions. Our findings indicate that continuous updating can help mitigate algorithmic discrimination and enhance economic efficiency over time. Importantly, we provide evidence that human decision makers in the loop who possess the authority to override ML predictions may impede the self-correction of discriminatory models and even induce initially unbiased models to become discriminatory with time. These findings underscore the complex sociotechnological nature of algorithmic discrimination and highlight the role that humans play in addressing it when ML models undergo continuous updating. Our results have important practical implications, especially considering the impending regulations mandating human involvement in ML-supported decision-making processes.
Recommended Citation
Bauer, Kevin; Heigl, Rebecca; Hinz, Oliver; and Kosfeld, Michael
(2024)
"Feedback Loops in Machine Learning: A Study on the Interplay of Continuous Updating and Human Discrimination,"
Journal of the Association for Information Systems, 25(4), 804-866.
DOI: 10.17705/1jais.00853
Available at:
https://aisel.aisnet.org/jais/vol25/iss4/9
DOI
10.17705/1jais.00853
When commenting on articles, please be friendly, welcoming, respectful and abide by the AIS eLibrary Discussion Thread Code of Conduct posted here.