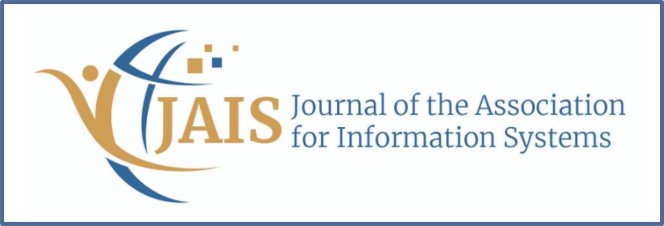
Abstract
Data-driven data science challenges our conceptualization of “data.” Significantly beyond capturing a given phenomenon, data, increasingly, are the phenomenon. Data may be iteratively manipulated algorithmically, undermining the “faithfulness” of data to any originating phenomenon. Crucially, data that are not “faithful” are inherently uncertain, as data risk becoming meaningless symbols. We empirically study how a community of commercially based geoscientists grapples with the phenomenon of offshore oil and gas reservoirs located kilometers below the seabed. The data available about these reservoirs are algorithmically manipulated sensor-based Internet of Things data. Our main contribution is the articulation of three patterns of work practices detailing how inherently uncertain data are woven into consequential work practices: (1) Accumulating is the cumulative process of supporting and triangulating one set of data with supplementary data sets; accumulating captures the conservative approach of backing up existing interpretations of the data. (2) Reframing describes the process where existing interpretations are contested by new data or models; reframing captures that there are limits to how far data can be manipulated. (3) Prospecting, or the cultivation of competing, incompatible data interpretations; while the former two patterns essentially attempt to regulate uncertainty, prospecting is about embracing it. Our concept of data-centric knowing is constituted by these three interwoven, ongoing practices.
Recommended Citation
Mikalsen, Marius and Monteiro, Eric
(2021)
"Acting with Inherently Uncertain Data: Practices of Data-Centric Knowing,"
Journal of the Association for Information Systems, 22(6), 1715-1735.
DOI: 10.17705/1jais.00722
Available at:
https://aisel.aisnet.org/jais/vol22/iss6/3
DOI
10.17705/1jais.00722
When commenting on articles, please be friendly, welcoming, respectful and abide by the AIS eLibrary Discussion Thread Code of Conduct posted here.