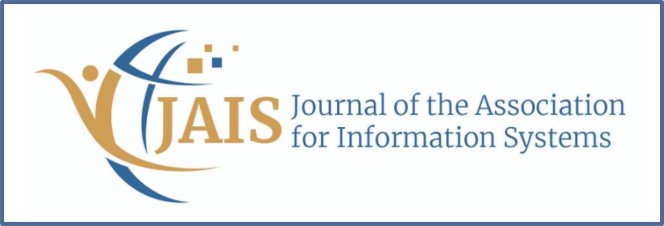
Abstract
We address the problem of recommending online communities on social media platforms using design science. Our method is grounded in network science and leverages the random surfer model of the web, small-world networks, strength of weak connections, and connectivity to analyze three types of large-scale networks. In doing so, we design features for structural hole assortativity and local clustering coefficient rank to capture both the diversity and evolution of user interests. We also extract general online community features such as size and overlap. Experiments conducted on a large dataset of 34,000 lists created and subscribed to by 1,600 active Twitter users over a six-month period showed that our network features outperform the general and content features in terms of recommending communities at the top position. In addition, a combination of general and network features generated the best results in the top position with a significant performance improvement over using only the content features. A combination of all three types of features gave the best results in the top-5 and top-10 positions while improving the quality of recommendations at every other position. Our work outperforms the latest work on community recommendations on social media platforms and has major implications for the design of online community recommenders.
Recommended Citation
Velichety, Srikar and Ram, Sudha
(2021)
"Finding a Needle in the Haystack: Recommending Online Communities on Social Media Platforms Using Network and Design Science,"
Journal of the Association for Information Systems, 22(5), 1285-1310.
DOI: 10.17705/1jais.00694
Available at:
https://aisel.aisnet.org/jais/vol22/iss5/7
DOI
10.17705/1jais.00694
When commenting on articles, please be friendly, welcoming, respectful and abide by the AIS eLibrary Discussion Thread Code of Conduct posted here.