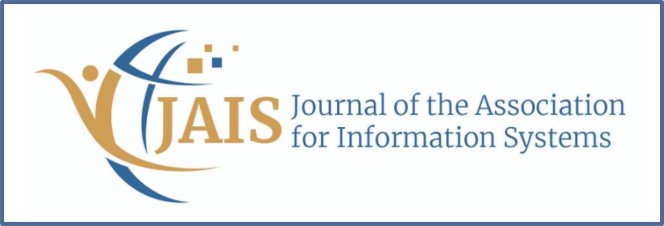
Abstract
We address the challenge of building an automated fraud detection system with robust classifiers that mitigate countermeasures from fraudsters in the field of information-based securities fraud. Our work involves developing design principles for robust fraud detection systems and presenting corresponding design features. We adopt an instrumentalist perspective that relies on theory-based linguistic features and ensemble learning concepts as justificatory knowledge for building robust classifiers. We perform a naive evaluation that assesses the classifiers’ performance to identify suspicious stock recommendations, and a robustness evaluation with a simulation that demonstrates a response to fraudster countermeasures. The results indicate that the use of theory-based linguistic features and ensemble learning can significantly increase the robustness of classifiers and contribute to the effectiveness of robust fraud detection. We discuss implications for supervisory authorities, industry, and individual users.
Recommended Citation
Siering, Michael; Muntermann, Jan; and Grčar, Miha
(2021)
"Design Principles for Robust Fraud Detection: The Case of Stock Market Manipulations,"
Journal of the Association for Information Systems, 22(1), .
DOI: 10.17705/1jais.00657
Available at:
https://aisel.aisnet.org/jais/vol22/iss1/4
DOI
10.17705/1jais.00657
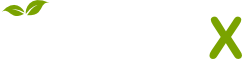
- Citations
- Citation Indexes: 20
- Usage
- Abstract Views: 2706
- Downloads: 726
- Captures
- Readers: 69
- Mentions
- Blog Mentions: 1
- News Mentions: 4
When commenting on articles, please be friendly, welcoming, respectful and abide by the AIS eLibrary Discussion Thread Code of Conduct posted here.