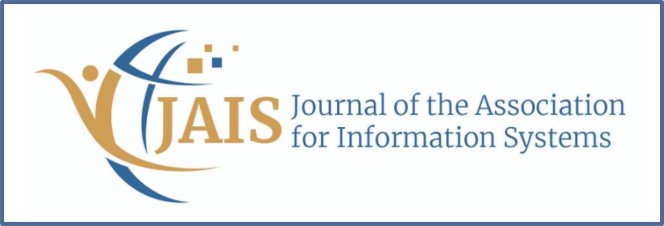
Abstract
This study concerns the risks of privacy disclosure when sharing and releasing a dataset in which each individual may be associated with multiple records. Existing data privacy approaches and policies typically assume that each individual in a shared dataset corresponds to a single record, leading to an underestimation of the disclosure risks in multiple records per person scenarios. We propose two novel measures of privacy disclosure to arrive at a more appropriate assessment of disclosure risks. The first measure assesses individual-record disclosure risk based upon the frequency distribution of individuals’ occurrences. The second measure assesses sensitive-attribute disclosure risk based upon the number of individuals affiliated with a sensitive value. We show that the two proposed disclosure measures generalize the well-known k-anonymity and l-diversity measures, respectively, and work for scenarios with either a single record or multiple records per person. We have developed an efficient computational procedure that integrates the two proposed measures and a data quality measure to anonymize the data with multiple records per person when sharing and releasing the data for research and analytics. The results of the experimental evaluation using real-world data demonstrate the advantage of the proposed approach over existing techniques for protecting privacy while preserving data quality.
Recommended Citation
Kartal, Hasan B. and Li, Xiao-Bai
(2020)
"Protecting Privacy When Sharing and Releasing Data with Multiple Records per Person,"
Journal of the Association for Information Systems, 21(6), .
DOI: 10.17705/1jais.00643
Available at:
https://aisel.aisnet.org/jais/vol21/iss6/8
DOI
10.17705/1jais.00643
When commenting on articles, please be friendly, welcoming, respectful and abide by the AIS eLibrary Discussion Thread Code of Conduct posted here.