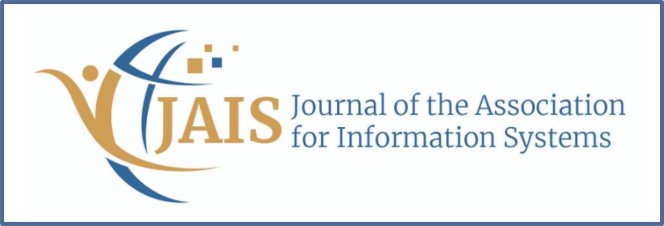
Abstract
Linear regression is among the most popular statistical models in social sciences research, and researchers in various disciplines use linear probability models (LPMs)—linear regression models applied to a binary outcome. Surprisingly, LPMs are rare in the IS literature, where researchers typically use logit and probit models for binary outcomes. Researchers have examined specific aspects of LPMs’ but not thoroughly evaluated their practical pros and cons for different research goals under different scenarios. We perform an extensive simulation study to evaluate the advantages and dangers of LPMs, especially with respect to big data, which is now common in IS research. We evaluate LPMs for three common uses of binary outcome models: inference and estimation, prediction and classification, and selection bias. We compare its performance to logit and probit under different sample sizes, error distributions, and more. We find that coefficient directions, statistical significance, and marginal effects yield results similar to logit and probit. In addition, LPM estimators are consistent for the true parameters up to a multiplicative scalar. This scalar, although rarely required, can be estimated assuming an appropriate error distribution. For classification and selection bias, LPMs are on par with logit and probit models in terms of class separation and ranking and is a viable alternative in selection models. LPMs are lacking when the predicted probabilities are of interest because predicted probabilities can exceed the unit interval. We illustrate some of these results by modeling price in online auctions using data from eBay.
Recommended Citation
Chatla, Suneel Babu and Shmueli, Galit
(2017)
"An Extensive Examination of Regression Models with a Binary Outcome Variable,"
Journal of the Association for Information Systems, 18(4), .
DOI: 10.17705/1jais.00455
Available at:
https://aisel.aisnet.org/jais/vol18/iss4/1
DOI
10.17705/1jais.00455
When commenting on articles, please be friendly, welcoming, respectful and abide by the AIS eLibrary Discussion Thread Code of Conduct posted here.