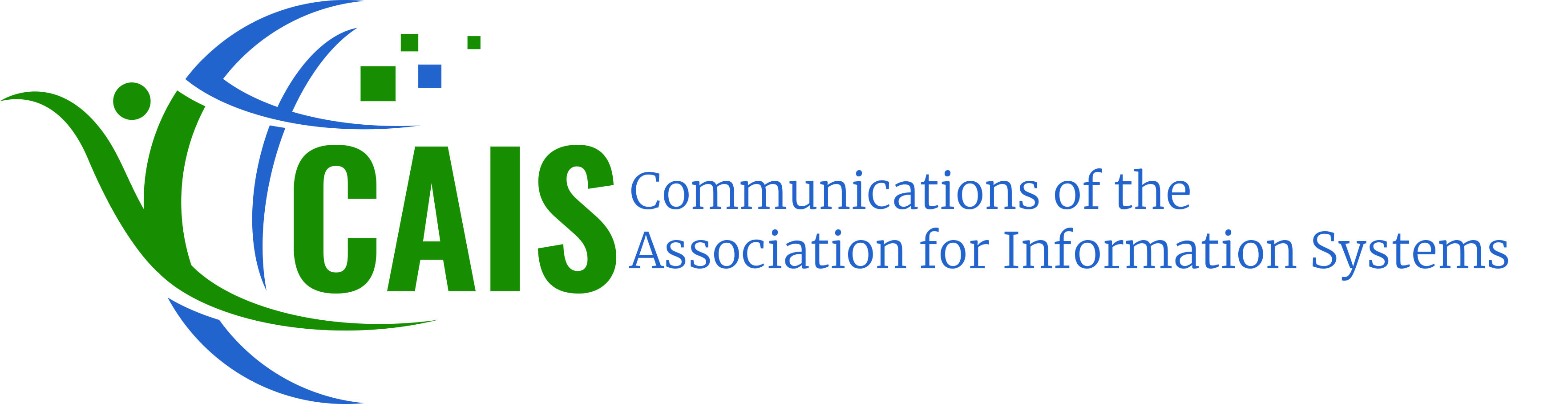
Author ORCID Identifier
Pei-Fang Hsu:0000-0002-3973-1770
Shih-Chu Chen:0000-0002-7561-6788
Wen-Yang He:0009-0001-1970-0723
Abstract
Survey is popularly used in conducting research. With the recent rise of big data, survey scholars are calling for integrating data mining methods into traditional survey studies to refine theories and exploit insights in new contexts. However, the integration of survey and data mining techniques to refine theories is still underdeveloped, and the importance of addressing the interpretability and non-interpretability of various data mining algorithms is greatly needed. To bridge these gaps, we integrate data mining predictions into a traditional survey-based study, demonstrating interpretable and uninterpretable algorithms that predict an individual's willingness to pay for e-learning markets. We provide a step-by-step guideline, from goal definition and data preparation to variable choice, prediction accuracy, and theory refinement, to assist survey scholars in combining the two research methods to enhance a well-regarded theory, the Technology Adoption Model (TAM). We discuss the trade-offs that survey scholars may encounter when combining the two methods in refining theories and suggest how they might overcome these inherent difficulties. By demonstrating a theory’s potential for improving prediction accuracy and exemplifying how data mining results can refine theories, our study contributes to survey scholars on how to refine theories to increase theoretical contributions with new data mining methods.
DOI
10.17705/1CAIS.05418
Recommended Citation
Hsu, P., Chen, S., & He, W. (2024). Data Mining Augmented Survey Research. Communications of the Association for Information Systems, 54, 491-518. https://doi.org/10.17705/1CAIS.05418
When commenting on articles, please be friendly, welcoming, respectful and abide by the AIS eLibrary Discussion Thread Code of Conduct posted here.