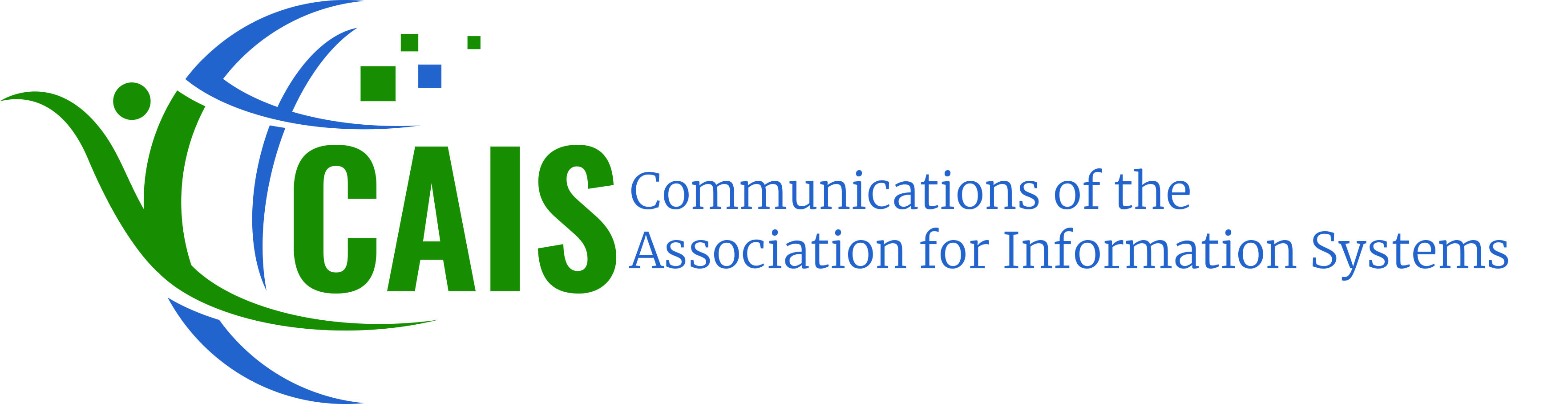
Abstract
This paper makes a focused methodological contribution to the information systems (IS) literature by introducing a bivariate dynamic latent difference score model (BDLDSM) to simultaneously model change trajectories, dynamic relationships, and potential feedback loops between predictor and outcome variables for longitudinal data analysis. It will be most relevant for research that aims to use longitudinal data to explore longitudinal theories related to change. Commonly used longitudinal methods in IS research – linear unobserved effects panel data models, structural equation modeling (SEM), and random coefficient models – largely miss the opportunity to explore rate of change, dynamic relationships, and potential feedback loops between predictor and outcome variables while incorporating change trajectories, which are critical for longitudinal theory development. Latent growth models help address change trajectories, but still prevent researchers from using longitudinal data more thoroughly. For instance, these models cannot be used for examining dynamic relationships or feedback loops. BDLDSM allows IS researchers to analyze change trajectories, understand rate of change in variables, examine dynamic relationships between variables over time, and test for feedback loops between predictor and outcome variables. The use of this methodology has the potential to advance theoretical development by enabling researchers to exploit longitudinal data to test change-related hypotheses and predictions rigorously. We describe the key aspects of various longitudinal techniques, provide an illustration of BDLDSM on a healthcare panel dataset, discuss how BDLDSM addresses the limitations of other methods, and provide a step-by-step guide, including Mplus code, to develop and conduct BDLDSM analyses.
DOI
10.17705/1CAIS.05018
Recommended Citation
Tao, Y., Mishra, A., Masyn, K. E., & Keil, M. (2022). Addressing Change Trajectories and Reciprocal Relationships: A Longitudinal Method for Information Systems Research. Communications of the Association for Information Systems, 50, pp-pp. https://doi.org/10.17705/1CAIS.05018
When commenting on articles, please be friendly, welcoming, respectful and abide by the AIS eLibrary Discussion Thread Code of Conduct posted here.