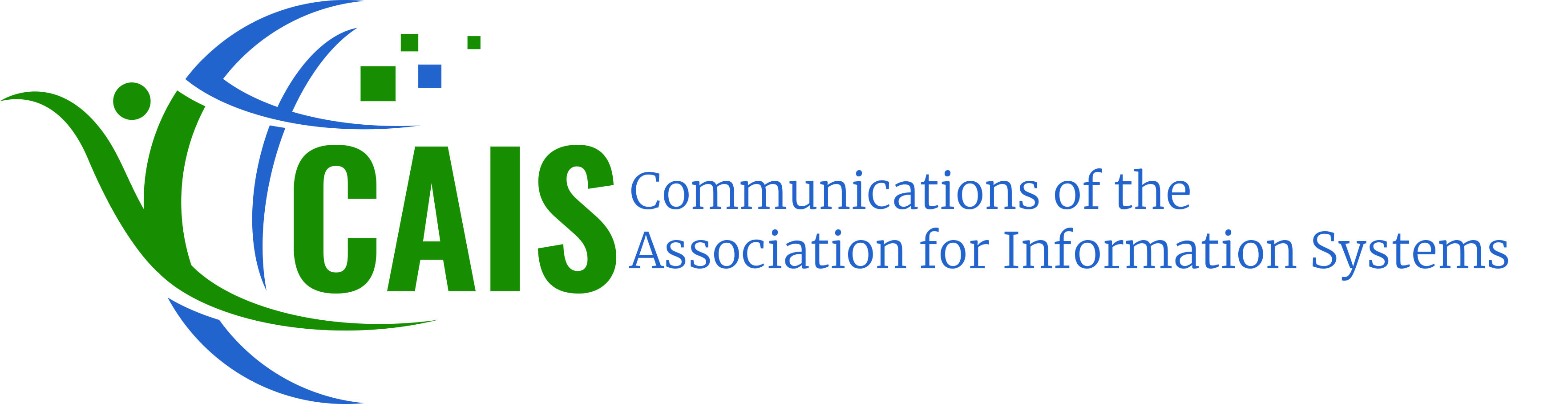
Abstract
The increase in the number of mobile devices that use the Android operating system has attracted the attention of cybercriminals who want to disrupt or gain unauthorized access to them through malware infections. To prevent such malware, cybersecurity experts and researchers require datasets of malware samples that most available antivirus software programs cannot detect. However, researchers have infrequently discussed how to identify evolving Android malware characteristics from different sources. In this paper, we analyze a wide variety of Android malware datasets to determine more discriminative features such as permissions and intents. We then apply machine-learning techniques on collected samples of different datasets based on the acquired features’ similarity. We perform random sampling on each cluster of collected datasets to check the antivirus software’s capability to detect the sample. We also discuss some common pitfalls in selecting datasets. Our findings benefit firms by acting as an exhaustive source of information about leading Android malware datasets.
DOI
10.17705/1CAIS.04626
Recommended Citation
Sharma, S., Kumar, N., Kumar, R., & Krishna, C. (2020). The Paradox of Choice: Investigating Selection Strategies for Android Malware Datasets Using a Machine-learning Approach. Communications of the Association for Information Systems, 46, pp-pp. https://doi.org/10.17705/1CAIS.04626
When commenting on articles, please be friendly, welcoming, respectful and abide by the AIS eLibrary Discussion Thread Code of Conduct posted here.