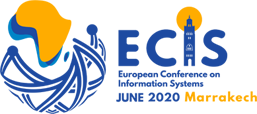
ECIS 2020 Research Papers
Abstract
The flexibility of general-purpose sentiment dictionaries has led to their extensive application in many different research fields. While these sentiment dictionaries are easy to apply, they are typically inferior to text classification approaches based on machine learning or compared to domain-specific dictionaries. Nevertheless, both approaches generally come along with additional manual data analysis. We address this problem by extending a domain-specific sentiment dictionary utilizing regularized linear models. We induce a dictionary extension that is trained on an extensive dataset and therefore particularly fitted for its specific purpose but can also straightforwardly be applied due to easy-to-use polarity word lists in both research and industry use cases. We develop this dictionary extension based on nearly 15,000 reports from financial analysts and demonstrate that the dictionary measures the sentiment of financial analysts more accurately than other finance-specific (but more general-purpose) dictionaries. We thus contribute to an improved analysis of the sentiments of financial analysts, who are the subject of many research projects as well as highly respected financial experts. Further, we show that our approach realizes context specificity while avoiding extensive manual data analysis.
Recommended Citation
Palmer, Matthias; Roeder, Jan; and Muntermann, Jan, "TOWARDS AUTOMATED ANALYSIS OF FINANCIAL ANALYST COMMUNICATION: THE INDUCTION OF A DOMAIN-SPECIFIC SENTIMENT DICTIONARY" (2020). In Proceedings of the 28th European Conference on Information Systems (ECIS), An Online AIS Conference, June 15-17, 2020.
https://aisel.aisnet.org/ecis2020_rp/212
When commenting on articles, please be friendly, welcoming, respectful and abide by the AIS eLibrary Discussion Thread Code of Conduct posted here.