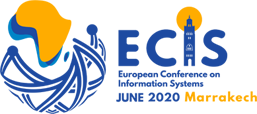
ECIS 2020 Research Papers
Abstract
In call center management, high forecast accuracy of arrivals’ predictions yielded by business analytics techniques is not only essential to enhance perceived service quality by shortening customers’ waiting times, but further to realize cost savings for companies by avoiding overstaffing. Thus, in this regard, methodological improvements are drivers for a company’s success within its competitive environment. Therefore, this research investigates the predictive impact of both lead times and model selection on accuracy. We analyze 174.5 weeks of data from an online retailer’s call center comprising customer support’s call and e-mail arrivals. We compare traditional as well as novel time series and machine learning (ML) models for ten different lead times with cross-validation and a rolling forecast origin. Our findings indicate that accuracy decreases with increasing lead time. Specifically, we prove that the models’ forecast types are impacted differently by lead times: For all lead time extents, regression and ML approaches maintain their accuracy levels with ex-post forecasts. In contrast, time series models’ ex-ante forecasts worsen the longer the lead time, i.e., the further the time series’ values used for prediction lie in the past. Overall, random forest (RF) outperforms the remaining models for almost all considered lead time constellations.
Recommended Citation
Rausch, Theresa Maria and Albrecht, Tobias, "The Impact of Lead Time and Model Selection on the Accuracy of Call Center Arrivals' Forecasts" (2020). In Proceedings of the 28th European Conference on Information Systems (ECIS), An Online AIS Conference, June 15-17, 2020.
https://aisel.aisnet.org/ecis2020_rp/19
When commenting on articles, please be friendly, welcoming, respectful and abide by the AIS eLibrary Discussion Thread Code of Conduct posted here.