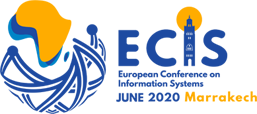
ECIS 2020 Research Papers
Abstract
Advances in the area of AI systems lead to the application of complex deep neural networks (DNN) that outperform other algorithms in critical applications like predictive maintenance, healthcare or autonomous driving. Unfortunately, the properties that render them so successful also lead to vulnerabilities that can make them the subject of adversarial attacks. While these systems try to mimic human behavior when transforming large amounts of data into decision recommendations, they remain black-box models so that humans often fail to detect adversarial behavior patterns in the model training process. Therefore, we derive a taxonomy from an extensive literature review to structure the knowledge of possible attack and defense patterns to create a basis for the analysis and implementation of AI security for scientists and practitioners alike. Furthermore, we use the taxonomy to expose the most common attack pattern and, in addition, we demonstrate the application of the taxonomy by projecting two real-world cases onto the taxonomy space and discuss applicable attack and defense patterns.
Recommended Citation
Heinrich, Kai; Graf, Johannes; Chen, Ji; Laurisch, Jakob; and Zschech, Patrick, "FOOL ME ONCE, SHAME ON YOU, FOOL ME TWICE, SHAME ON ME: A TAXONOMY OF ATTACK AND DE-FENSE PATTERNS FOR AI SECURITY" (2020). In Proceedings of the 28th European Conference on Information Systems (ECIS), An Online AIS Conference, June 15-17, 2020.
https://aisel.aisnet.org/ecis2020_rp/166
When commenting on articles, please be friendly, welcoming, respectful and abide by the AIS eLibrary Discussion Thread Code of Conduct posted here.