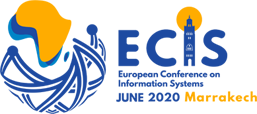
ECIS 2020 Research Papers
Abstract
Multi-label document classification is a common task and has become increasingly important for current business needs. However, generating keywords is not easily done as, next to methodological challenges, labeled training data for supervised classification does not always exist in the desired amount or quality. Therefore, methods that do not require labeled training data (e.g., unsupervised learning or statistical approaches) are valuable for practice. As none of these approaches alone provides optimal results in terms of recall and precision, we show that it is worth examining existing approaches for complementary strengths in order to combine them. We found such complementary strengths for an unsupervised word embedding method and the term frequency–inverse document frequency method (tfidf) and propose a combined approach. For evaluation, we test the combined approach on a data set from a public broadcaster in Germany and show that recall and precision can be significantly improved.
Recommended Citation
Hirschmeier, Stefan; Melsbach, Johannes Werner; Schoder, Detlef; and Stahlmann, Sven, "Improving Recall and Precision in Unsupervised Multi-Label Document Classification Tasks by Combining Word Embeddings with TF-IDF" (2020). In Proceedings of the 28th European Conference on Information Systems (ECIS), An Online AIS Conference, June 15-17, 2020.
https://aisel.aisnet.org/ecis2020_rp/99
When commenting on articles, please be friendly, welcoming, respectful and abide by the AIS eLibrary Discussion Thread Code of Conduct posted here.