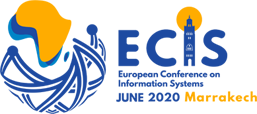
ECIS 2020 Research Papers
Abstract
The proliferation of enterprise information systems allows to capture the digital footprints generated over various user interaction phases. Such transaction data describe the details of the user interactions and the underlying processes on a fine granular level. Building capabilities to analyse the transactional process data is a key success differentiation factor that enables to grasp the user behavior more effectively. In this study, we aim to propose a prescriptive process analytics approach by combining the approaches from the machine learning, process mining and explainable artificial intelligence (XAI) research domains. After examining predictability of the business processes by employing an advanced deep learning approach, this study applies for the first time both in the business process prediction and customer journey analytics research domains an XAI technique, Partial Dependence Plots (PDP), to generate causal explanations. The real-life process data delivered by various information systems of a Dutch autonomous administrative authority were used to investigate the appropriateness of the proposed prescriptive analytics approach. The applied deep learning approach achieves a very good performance with an Area Under ROC Curve of 0.933. The generated explanations with PDP give insights to identify a set of alternative courses-of-actions to prevent the undesired outcomes.
Recommended Citation
Mehdiyev, Nijat and Fettke, Peter, "PRESCRIPTIVE PROCESS ANALYTICS WITH DEEP LEARNING AND EXPLAINABLE ARTIFICIAL INTELLIGENCE" (2020). In Proceedings of the 28th European Conference on Information Systems (ECIS), An Online AIS Conference, June 15-17, 2020.
https://aisel.aisnet.org/ecis2020_rp/122
When commenting on articles, please be friendly, welcoming, respectful and abide by the AIS eLibrary Discussion Thread Code of Conduct posted here.