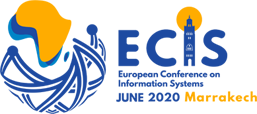
ECIS 2020 Research Papers
Loading...
Abstract
Anomaly detection in large scale accounting data is one of the long-standing challenges in the financial audit practice. Accounting professionals have therefore resorted to advanced machine learning techniques to address this. Although being quite successful, existing supervised and unsupervised anomaly detection algorithms come with certain drawbacks. In order to overcome them, an innovative semi-supervised machine learning approach is proposed in this paper which combines both unsupervised and supervised algorithms for anomaly detection in big data. The unsupervised algorithm, i.e. DBSCAN is first applied on a representative subset of the data to generate a training set based on pseudo labels of anomalies. Afterward, the training set is used to direct the supervised algorithm, i.e. LightGBM for anomaly detection in the remaining data. This approach is applied to an insurance policy dataset consisting of approximately 32 million records. Our proposed framework helps capture 90% and 96% of anomalous observations by investigating 5% and 10% of the data respectively. Comprehensive details are provided throughout the paper to present the practical applicability and wide-spread potential of the proposed semi-supervised approach for similar problem categories.
Recommended Citation
Bhattacharya, Indranil and Roos Lindgreen, Edo, "A SEMI-SUPERVISED MACHINE LEARNING APPROACH TO DETECT ANOMALIES IN BIG ACCOUNTING DATA" (2020). In Proceedings of the 28th European Conference on Information Systems (ECIS), An Online AIS Conference, June 15-17, 2020.
https://aisel.aisnet.org/ecis2020_rp/100
When commenting on articles, please be friendly, welcoming, respectful and abide by the AIS eLibrary Discussion Thread Code of Conduct posted here.