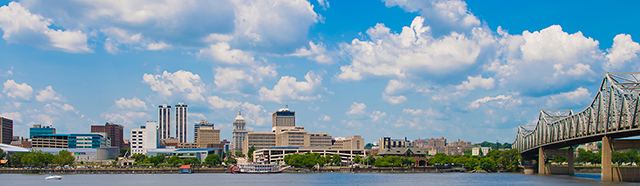
MWAIS 2024 Proceedings
Abstract
This research study aims to identify major mechanisms through which machine learning (ML) and organizational learning coevolve. Organizational learning (OL) involves creating, retaining, and transferring knowledge. The organizational learning journey entails experience accumulation and, thus, modification of routines. The prevalence of machine learning (i.e., AI tools) in business processes, products, and services necessitates new perspectives on how OL and ML interact when (1) ML tools coexist with the current routines and human decision-making (e.g., AI stylists at Stich Fix), (2) ML tools replace current routines and human decision-making processes (e.g., predictive maintenance models at C3.ai). Building upon Nonaka’s model of knowledge management (Nonaka & Takeuchi, 1995) and the extant literature on AI impacts on organizational learning, we plan to develop an ML/OL coevolution model that’s anchored in the following three dimensions: (1) locus of knowledge, (2) locus of agency in protocol development, and (3) locus of agency in action selection (Murray et al., 2021). The literature suggests that the full benefits of machine learning are realized only when organizations can learn with AI and are willing to evolve their existing routines accordingly. We draw upon the literature on organizational learning, IT-enabled knowledge management, and human-machine interaction to investigate the mechanisms of ML and OL coevolution, including its enablers and obstacles. We also plan to revisit the classic concepts of tacit and explicit knowledge, the exploration-exploitation trade-off, organization memory, knowledge decay, and unlearning to unravel how these mechanisms will be interwoven with ML processes. The intended contributions are a theoretical framework and a set of propositions. We aim to delineate specific mechanisms through which distinct ML types interact with sub-processes such as knowledge creation, retention, updating, forgetting, and transfer.
Recommended Citation
Javadi, Elahe and Gebauer, Judith, "From Machine Learning to Organizational Learning" (2024). MWAIS 2024 Proceedings. 21.
https://aisel.aisnet.org/mwais2024/21